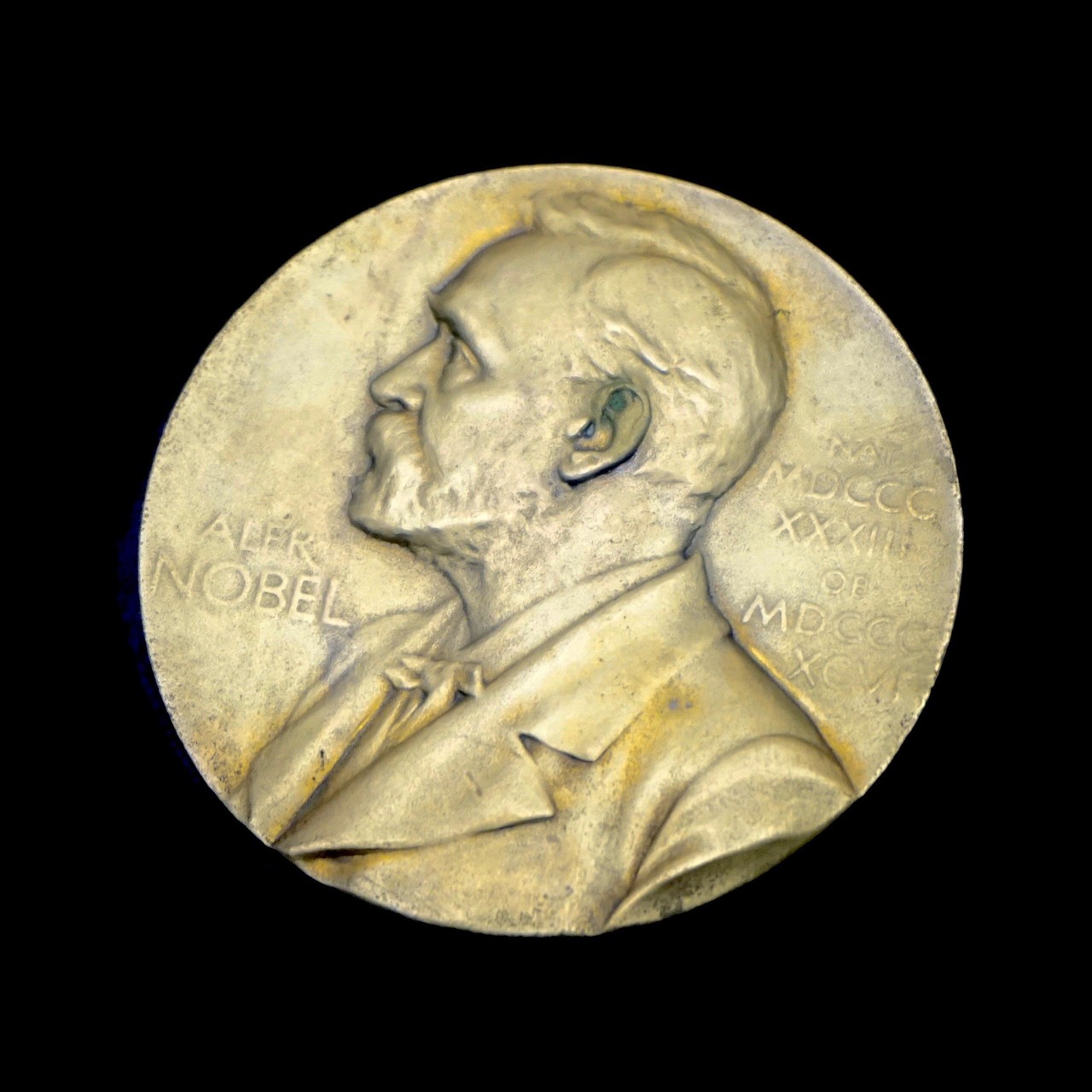
The 2024 Nobel Prize in Chemistry: A simple explanation
The 2024 Nobel prize in Chemistry went to David Baker, Demis Hassabis and John Jumper, the three men behind cracking the 50-year-old problem of predicting proteins’ structures. Now, if you are anything like me, you might be intrigued to know more about how these three scientists worked and what the problem they addressed was all about. After all, a Nobel Prize isn’t awarded every day. The following article should help familiarize you with their work and what led them to take this project on.
The world is becoming increasingly curious about complex biostructures. As the World Economic Forum put it: “The biorevolution is kicking off” 1 .This means that significant biological progress is being made, attracting experts and scientists from a variety of fields. Many of the Nobel Prizes awarded in chemistry over the past 20 years were on biochemistry or used chemical knowledge to solve complex biological problems.
A Nobel prize problem –Why are protein structures problematic?
If you are a bit familiar with high school biology, then you’ve heard about proteins and amino acids: but what does this have to do with the 2024 Nobel prize?
In essence, sequences of your DNA ‘code’ for a specific amino acid. For example the DNA sequence ‘UCU’ codes for the amino acid serine. There are around 20 different types of amino acids, each one being a little different from the other.
Amino acids bind to each other and eventually form a protein. This is why the specific DNA ‘codes’ and the order they are found in greatly impacts the protein’s final function. But serine has different properties from e.g. arginine and if they happen to bind to each other, they will interact with each other and form a different protein from, say, serine and histidine. So pretty much, many of your proteins are determined by your DNA. Let’s dive a bit deeper into this:
Let’s look at this example: the DNA sequence ‘GGA’ codes for the amino acid ‘Glycine’. This Glycine molecule bonds with other amino acids. This forms a long chain of amino acids, which is what we call a polypeptide. This polypeptide goes on to twist and bend itself. This is where the properties of amino acids come into play. Based on how the different amino acids interact with each other, the polypeptide will twist differently.

This polypeptide, now arranged in alpha helix or beta sheets, will fold into a protein which will take on a specific function. Proteins are responsible for a large amount of biochemical processes. From catalyzing your metabolism to being in your food.
Depending on the sequence of the amino acids, the polypeptide will be coded to have different functions. This will cause it to behave uniquely once it assembles into a protein.
On a chemical level, amino acids are a combination of an amine group (NH2) that is bonded to a carbon atom and a carboxyl group (COOH). Thus making their structural formula: RCH(NH2)COOH where R represents an additional group that is bonded to the the carbon atom.
When amino acids bond to create a polypeptide, the COOH and the NH2 groups bond. This forms a H2O molecule that is consequently released. You can imagine this as a reaction happening between the two amino acids that would theoretically be described with the equation:
RCH(NH₂)COOH + RCH(NH₂)COOH → RCH(NH₂)CONHCH(R)COOH + H₂O
Since the polypeptide is essentially a very large molecule, writing an equation for it doesn’t make much sense, but this might clarify how the reaction happens.

The image above illustrates what a polypeptide chain looks like on a chemical level. Take notice of how the R groups, which vary according to amino acid, are located at the ‘bottom’ of the molecule next to each other. This plays a significant role in the folding of the polypeptide, as the R groups vary in their chemical nature. These R groups have different properties, as discussed earlier:
positive / negative charge
polarity / no polarity –> hydrophobic or hydrophilic (water repellent or not, which plays a significant role in an aqueous solution, such as your cell)
Hydrogen bonding plays a part in the assembly of alpha helixes and beta sheets.
These properties and the order in which amino acids are arranged, influences how the polypeptide will fold. For example, a positively charged R group will be attracted to a negatively charged R group of another amino acid. This will jerk the polypeptide into different geometric arrangements (in this case the two groups will be attracted to each other).
Polypeptides can have undefined lengths and the amino acids present in the polypeptide bond to each other in a random order. This means that proteins become extremely complex once folded a few times over. This is a key issue if we want to find out how each of these proteins are assembled.
Furthermore, genes can experience mutations that lead to denatured (not functional) or slightly altered proteins, thus complicating the process of determining protein structures further.
How does AI mix into the Nobel prize for chemistry?
With a solid understanding of why proteins assemble into complicated structures, let’s get back to the work behind our Nobel prize.
Determining protein structures was often done using X-ray crystallography. This process allows us to determine the position of atoms in a biochemical structure.
It is what Watson and Crick used to identify the double helix structure of the DNA molecule in 1953. However this technique is very difficult to master and due to its experimental nature can be very intricate.

Computers, on the other hand, are increasingly being used in biochemistry. 50 years ago, scientists would model biochemical structures using metal building blocks. Nowadays, everything is done using 3D modeling software that allows speed and efficiency.
AI, in particular, has the advantage of needing less code to do more action. This means that it can learn from its mistakes and thus become very good at something in much less time. Hence biologists have started to mix AI and computerization into many of the processes that were done by hand earlier. Makes sense, right?
This comes into play when talking about our laureates.
In 1994, CASP was launched (Critical Assessment of Structure Prediction). It was a worldwide experiment and worked a bit like a competition. Researchers would research and carefully predict the structures of proteins and ultimately submit them to the ‘competition’. These predictions were then compared to their actual structures and given a score out of 100.
CASP’s goal was to advance protein structure methodology and technology. Surely enough, in 2018 a participating group by the name of AlphaFold won CASP13.
AlphaFold is an AI program created by DeepMind, which was bought up by Google in 2014. Deepmind is also the creator of another AI program, AlphaGo. This program would beat a world-class player at the table game Go in 2017. But let’s get back to the chemistry.
AlphaFold made headlines when it won CASP13 and CASP14 with a score of around 90/100. DeepMind’s CEO, Demis Hassabis, along with two other scientists won the 2024 Nobel Prize in Chemistry for it, as mentioned before. These scientists came out of the fields of artificial intelligence and chemistry/biology.
Thus this poses one final question: how can AlphaFold predict protein structures with such mouth-gaping accuracy?
Intelligent algorithms used in biology and chemistry?
AlphaFold predicts protein structures by accessing a database of protein structures.
It scans this database for amino acid sequences that are similar to the input. For example, by taking the DNA code for a protein of a certain species and comparing it to the codes of other species. Multiple Sequence Alignment2 (MSA) is a tool used to compare these ‘codes’ to each other, as seen below. These similarities between codes tell us more about the protein.

By observing this matrix of different sequences and doing a comparison, you can learn which features of the sequence have been conserved and which have mutated in the different species. Think of evolution. If a protein kept certain features (represented through different amino acids) throughout many millions of years, these sequences may be more important than others to fulfill its function. Comparison thus provides information on which function the protein should fulfill.
Another way AlphaFold processes input is by comparing each element of the amino acid sequence to each other. By doing this, the program can practically determine how these amino acids will behave in an environment with each other (due to their charges, polarity… etc.). This helps the system determine e.g. the angle at which the amino acids are located to each other or even their distances. This is called pair representation.
Both the MSA and the pair representation, will be updated throughout the algorithm. This allows it to improve and deepen its understanding of the protein.
The MSA’s observations will update the pair representation’s and vice versa. For example, the pair representation might have detected a relationship between two amino acids, that the MSA can use to refine its own knowledge on that relationship. This is an underlying principle of machine learning. As you can see, the machine literally ‘learns from itself’.

(This post won’t delve too deeply into the exact workings of this, but there are some links at the end of the page if your interest holds. 3)
The MSA and pair representation’s goal is to create a 3D structure hypothesis of the different proteins.
In order to do this, both the MSA and pair representation will be updated a total of 48 times4 . Essentially, until the system is left with the refined versions of the MSA and pair representation, that can set up the most accurate 3D structure hypothesis. Once this is completed, a module, the structure module, generates a 3D protein structure based on the information collected in the steps before.
This modeled protein structure is fed into the MSA and pair representation again for a total of 4 times to improve the accuracy of the model.

Once a final model has been set up, AlphaFold essentially gives itself a rating on how good it did at determining the position of each amino acid in the protein structure. This can be seen when visiting the AlphaFold website and searching for a protein.
For example, you can see the predicted structure of the protein alpha-amylase A1 with the AlphaFold’s ‘rating’ (pLDDT score) on the position of the amino acids.

In this case AlphaFold’s rating is 98.79 (very high).
Conclusion – Not just a Nobel prize in chemistry, but a breakthrough?
So now you have acquired an understanding of how AlphaFold works and of what the work behind the 2024 Nobel Prize was all about. Let’s hope that programs such as AlphaFold can continue to make breakthroughs in fields such as biochemistry and precise their predictions of molecules. Especially interesting is the study of denatured proteins and mutations which are the cause for diseases such as Alzheimer’s or Parkinson’s.5 AI might really revolutionize the field of genetics.
Have you ever wondered how trees communicate with each other?
- World Economic Forum ↩︎
- Note: If you are interested in making your own MSA and comparing proteins of different species: CLUSTAL OMEGA . Use PubMed’s Gene library to find the DNA sequence of different species’ hemoglobin (or any other protein): PubMed ↩︎
- UV BIO has a detailed explanation of AlphaFold. Understanding Multiple Sequence Alignment OMICS tutorials ↩︎
- The Evoformer has 48 blocks. ↩︎
- An article explaining this by Nature.com ↩︎